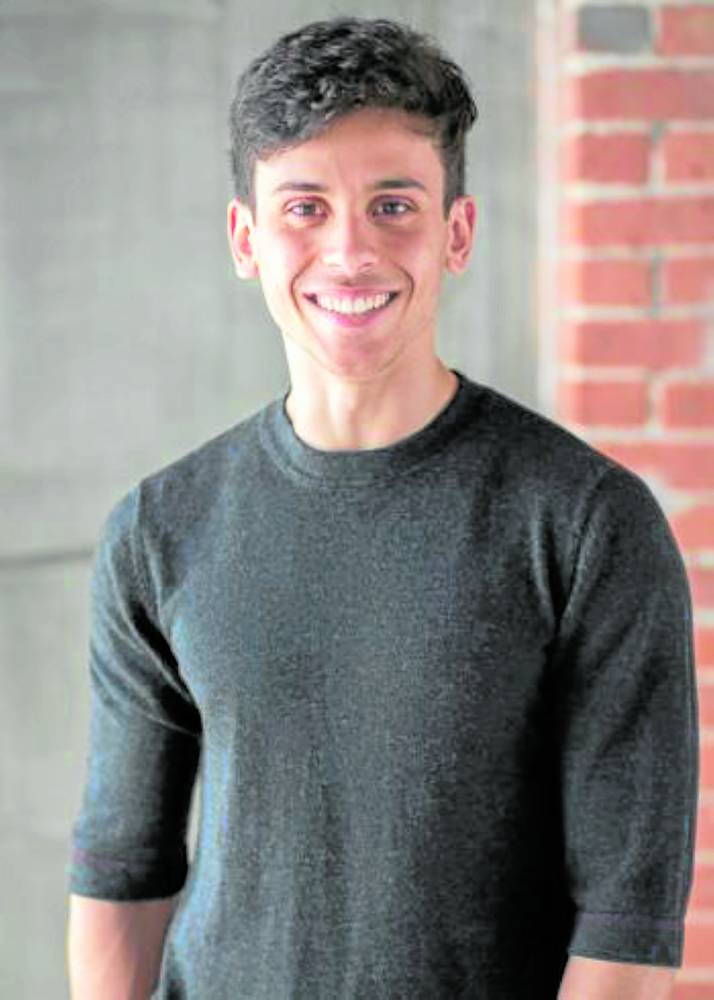
When it comes to cancer, we know that early detection saves lives. While there are gold-standard cancer screenings, cancer is often detected when it’s already growing or in its advanced stages. To catch the disease early, a professor at the Massachusetts Institute for Technology developed a robust artificial intelligence (AI) that can predict, with accuracy, future breast cancer occurrence.
Dr. Adam Yala, the scientist behind this medical study, developed an algorithm using a machine-learning approach. That means this technology imitates intelligent human behavior—it is constantly learning from data and then applying that learning without human intervention. Just like humans, its performance, based on a set of tasks, improves as it processes more and more data.
Unlike humans, though, machines register subtle changes with precision. The human eye cannot detect an ultra-fine change in the tissues influenced by age, genetics, hormones, weight or lactation. But it’s not just about minute changes that the human eye cannot see, but also about strained human eyes making errors in judgement after analyzing several images.
The online ads you see, for example, are based on your online behavior, the products you click or do not click. Just like how machines track patterns to show you products you will likely be interested to see, this robust AI maps the medical history of a multitude of patients to predict cancer or formulate better treatments.
Strategies and guidelines
“If you want to catch cancer early, you have two problems you need to solve. The first one is understanding, well, who’s going to get cancer in the near future,” Yala said during the Southeast Asian Breast Cancer Symposium (SEABCS) 2022. “Second problem is, how do we effectively create strategies and guidelines to catch cancer at the earliest stages possible and have the best possible treatment pathways.”
Yala was working on the algorithm called Mirai even before the pandemic. When COVID-19 happened, many cancer patients skipped checkups and scans out of fear of contracting the virus. The Massachusetts General Hospital devised a new approach to screening women for breast cancer—it started using the algorithm to predict who is at the most risk of developing cancer.
At that time, the AI accurately identified women at the most risk. (The hospital managed to convince these women to show up for their scans, and it turned out they had early signs of cancer.)
Mirai analyzes the standard mammogram and collects data about the patient, like the history of surgery or hormone factors. It was significantly more accurate than existing methods in predicting cancer risks and identifying high-risk groups. Mirai identified two times more future cancer diagnoses than the Tyrer-Cuzick model.
The Tyrer-Cuzick model is the current clinical standard tool that assess breast cancer risk. It is based on extensive information like age at first period, height, weight, childbearing history, family history, menopausal status, hormone replacement use.
“The traditional approach seen in clinics all over the world relies on expert clinical knowledge,” Yala said. “And the way we normally used images in this kind of clinical calculator domain is by summarizing them into a human-defined biomarker data that is fairly scattered and heterogeneous.”
The AI can make a big difference in this approach, he said. He said that a key AI task is to learn the association directly from the pixels of the image. The current one-size-fits-all approach is independent of health disparities. Different populations have different ages of onset cancer distributions. The AI model looks at someone’s risks and directly predicts when she or he should come back.
Best screening policy
Mirai works by putting a mammogram image through an “image encoder.” Each image, including the views it came from, is aggregated with other images from other views to obtain a representation of the entire mammogram. The mammogram typical comes with the Tyrer-Cuzick model. If that’s unavailable, predictive values are used. With these data, the additive “hazard layer” predicts a patient’s risk for breast cancer for each year over the next five years.
The additive “hazard layer” is a tool developed to address data that do not make sense, like a higher-risk patient that develops cancer in two years instead of five.
The AI reduces overtreatment, over-screening, and even false positives that lead to unnecessary anxiety and cost for patients who never develop breast cancer. It reduces screening where it’s not needed.
“And then we can optimize this machine-learning model to give us the best screening policy, a longitudinal perspective that’s across the entire population,” he said. “We can offer a continuum of different possible screening strategies. Regardless of how much screening we’re doing, we can do better than one-size-fits-all.”
Yala said they have done a lot of work globally to make sure it works for very different, diverse populations.
“Across all different settings with very different populations, you find the model achieves essentially similar or the same performance,” he said. “It means we can better devote our resources to high-risk screening. We need to understand who to prioritize for call-back for screenings.” —CONTRIBUTED